Recomb - Computational Proteomics
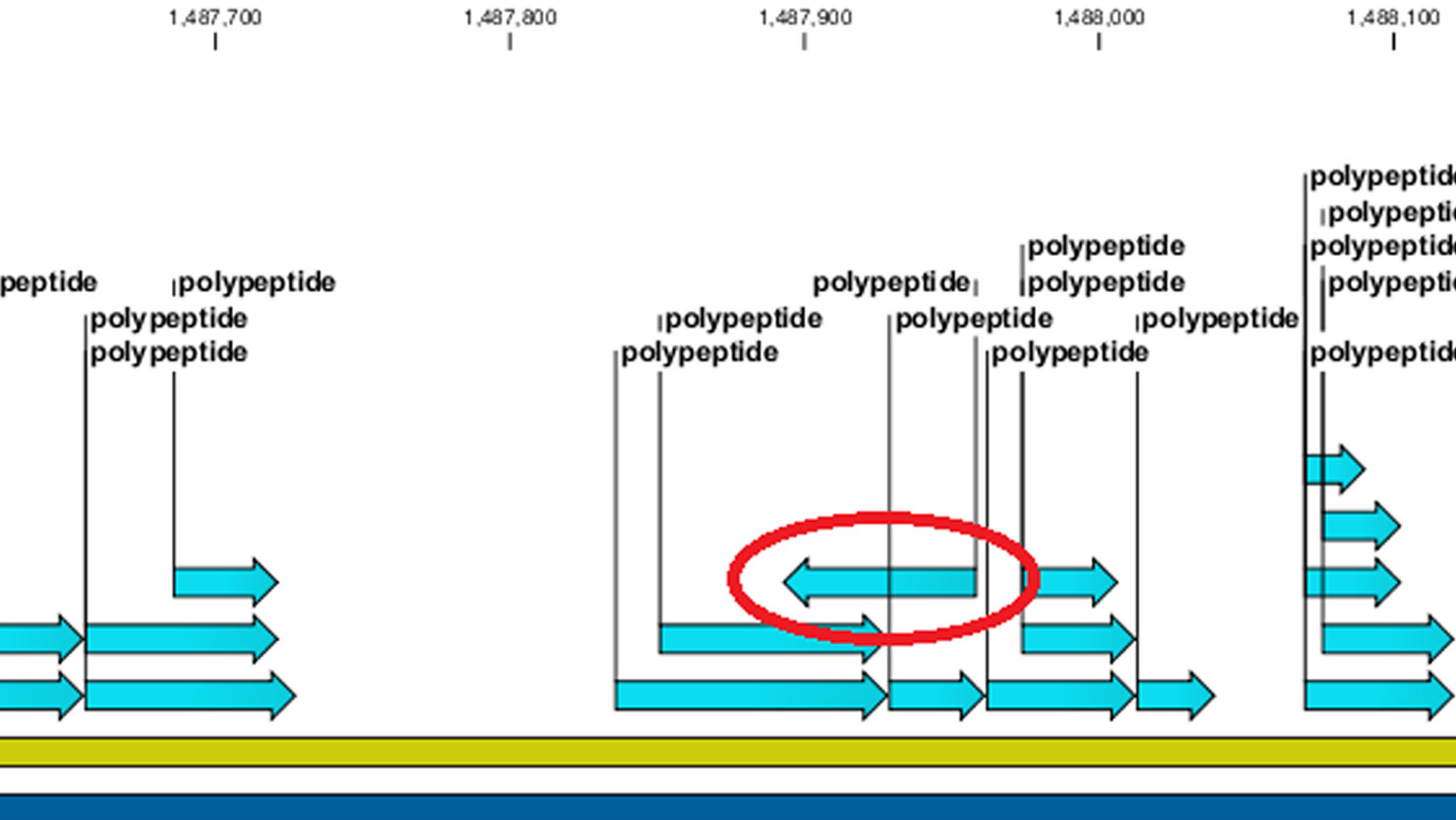
I recently attended the Recomb satellite conference on Computational Proteomics (downloads for talk and poster) in San Diego, CA. It was a kind of homecoming for me. I was a computational proteomics researcher at UCSD as a grad student with Vineet Bafna. Many of my classmates were still there, as were lots of familiar faces and friends. I joked with Marshall Bern, that this was almost all of the people at ASMS that I wanted to hear gathered in the same room, and it was only two days. Oh, and there was a beach.
Revisiting computational proteomics was a lot like hearing an old high school favorite on the radio. You sing along with the chorus and then mumble through the verse. But by the end, you remember it all again. Having diversified my research interests while at JCVI, I was excited to hear new developments in proteomics, as well as get a refresher on some oldies-but-goodies.
I presented the progress on my proteogenomics research, introducing some comparative proteogenomics. I submitted a poster abstract, and was asked to give a “flash-presentation” during the Saturday session as well. As the attendees were like-minded geeks, I presented my troubles spots as well as the highlights, hoping for advice and suggestions. For highlights, we have the pipeline running smoothly on the JCVI infrastructure, and can analyze any LTQ dataset rapidly. This produces a list of genomic loci needing re-annotation. I have somewhere around 10 datasets done now, and they all have been informative. Each shows a significant amount of gene novelty. As we amass a more diverse set of results, we are starting to look at the annotations from a comparative or evolutionary standpoint.
For trouble spots, I noticed that larger datasets need more attention to downstream processing. You can’t simply claim that all ‘novel’ peptides are new genes. First, we require high quality PSMs (p< 0.005 ) and then do a fairly detailed analysis of results. I found that even with very strict PSM filters, there were a significant number of false-positive ORF identifications. For this I have started to develop ORF level filters that look at the set of peptides in an ORF and not the individual ORF identification. You could, as Pavel suggested to me, go with perfect ‘zero FDR’ at the PSM level, but that tends to kill your spectrum identifications to the point where you see very few novel results (slide 6 from the talk). I feel that a more sensitive approach is to introduce the orthogonal filter (ORF level). I hope that later this summer I’ll be able to get out a Nature Methods paper about all the lessons learned. Till then …